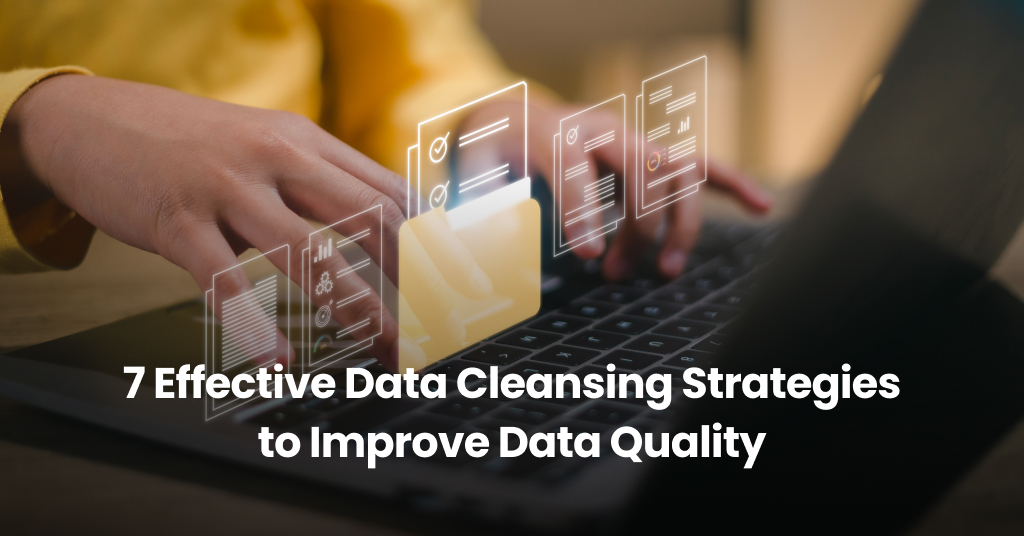
From small startups to large enterprises, every organization can benefit from maintaining high-quality data. Clean and reliable data paves the way for improved customer experiences, streamlined operations, better-targeted marketing campaigns, and enhanced overall business operations. On the other hand, poor data can have substantial consequences, such as:
- Inaccurate decision-making
- Reduced customer satisfaction
- Wasted resources and time
- Damaged reputation and credibility
- Inefficient business processes
- Missed growth opportunities
A survey conducted by Monte Carlo highlighted that businesses reported poor data quality, impacting 31% of their revenue. Therefore, keeping the data clean is critical to smooth business functioning.
Characteristics of good quality data
High-quality data possesses essential attributes that qualify it for decision-making and analysis. Let’s explore some key characteristics:
- Accuracy: It measures the correctness of the data and whether it is free from errors, inconsistencies, or biases that might impact its reliability.
- Completeness: Data completeness ensures that all required information has been collected and any missing values are identified and addressed to avoid gaps in the dataset.
- Consistency: It evaluates the degree to which data values conform to defined rules or standards, minimizing conflicts or discrepancies between different sources or versions of data.
- Reliability: Data is sourced from credible and authoritative channels, forming a solid foundation for well-informed conclusions and decisions.
- Relevance: It rates whether the data is appropriate and valuable for the intended analysis or purpose, ensuring that only pertinent information is considered.
- Timeliness: With up-to-date information, the data ensures relevance and avoids reliance on outdated or irrelevant records.
- Validity: Compliant with defined standards and formats, the data adheres to established guidelines and rules.
- Uniqueness: Minimal duplicates or redundancies guarantee efficient storage and prevent skewed analysis.
- Contextual Information: Accompanied by relevant metadata, the data provides vital context, enhancing its interpretability and usability.
Level up your data quality!
Let’s help you ensure a data-driven culture for informed decision-making, growth, and favorable business outcomes by learning some of the proven data cleansing strategies.
1. Effective data profiling
It involves analyzing the content, structure, and quality of your data to understand its characteristics and identify issues. Under profiling, you examine data statistics such as the number of missing and unique values, data types, or data distributions. This will help you recognize diverse aspects and highlight areas that require cleansing.
Example: In a customer database, data profiling may reveal that some customer records have missing phone numbers while others contain inconsistent address formats. Identifying these issues will allow you to resolve them effectively.
2. Standardization of data
Data collected from multiple sources or systems can vary in formats, units, and naming conventions. Therefore, it is essential to transform it into a unified structure that follows a standard set of rules and guidelines. Data standardization ensures that all data elements are presented in a consistent manner, eliminating discrepancies that can arise due to different data representations. Doing so reduces confusion, improves data quality, and facilitates easy comparison and analysis.
Example: In a sales dataset, standardizing product names and categories helps avoid ambiguities caused by spelling variations or abbreviations. For instance, “Apparel,” “Clothing,” and “Clothes” can be standardized into a single category, “Clothing.”
3. Removing redundancies
Data might be prone to duplicate records for various reasons, such as data entry errors, system glitches, or merging of multiple datasets. These duplicates can lead to inaccurate analysis, biased insights, or inefficiencies in data processing. With deduplication, you can identify and eliminate redundancies to eliminate data clutter, enhance accuracy, and prevent overrepresentation in statistical analysis.
Example: A mailing list database accumulates duplicate entries for the same individual due to new sign-ups and updates; recipients with multiple records may receive duplicate promotional emails or newsletters. This redundancy can lead to annoyance and potential unsubscribing from the mailing list.
4. Handling missing values
Missing values in a dataset can occur due to various reasons, such as human error or incomplete data collection. They can significantly impact the dataset’s quality and may lead to biased or erroneous conclusions if not addressed properly. Proper handling of missing values involves implementing multiple techniques (mean, median, or regression imputation) and considerations to maintain data integrity. Since this requires technical expertise, you can always leverage assistance from an experienced data cleansing company.
Example: In a survey dataset, if respondents fail to answer specific questions, certain methods like mean imputation (replacing missing values with the mean of that attribute) or predictive imputation (using regression models to predict missing values) can be employed to handle the data gaps.
5. Validating and verifying
Validation or error checking is an integral component of data quality assurance and cleansing processes. It involves verifying the accuracy, completeness, and consistency of data by applying predefined rules or checks. The goal is to ensure data adheres to specified criteria, constraints, and business rules.
Example: If an eCommerce company maintains a database of customer orders, including customer names, order dates, quantities, or prices, they can apply a range check to ensure that the quantity of products ordered falls within a reasonable range. For instance, it may be static, so the quantity must be between 1 and 100 units per product.
6. Detecting outliers
Outliers are data points that significantly deviate from the majority of the dataset. These anomalies can distort statistical analysis and adversely impact the accuracy of predictive models. They can be removed, transformed, and managed with robust methods to improve data accuracy for better decision-making and insight gathering.
Example: Upon analyzing the sales revenue data, an eCommerce company notices a significant outlier in June where the revenue reaches an unusually high value of $1,200,000. After further investigation, it was discovered that the spike in revenue for June was due to a one-time bulk order from a corporate client. Therefore, the company opts for a more accurate representation of regular sales patterns, leading to better predictions and informed business strategies.
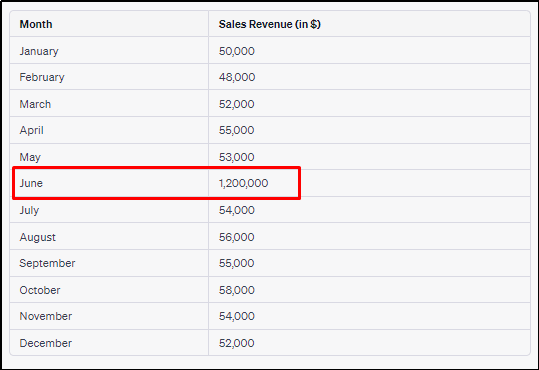
7. Integration and cross-referencing
Data integration combines data from various sources into a cohesive and unified dataset. Cross-referencing, on the other hand, involves linking related data to ensure consistency and completeness. These processes are vital to creating a comprehensive and accurate dataset for analysis.
Example: A medical organization aims to improve patient care and research outcomes by integrating health records from multiple departments. Each department maintains separate electronic health records (EHRs) containing patient demographics, medical history, lab results, or treatment information. To create a comprehensive patient database, the organization integrates the EHRs from various departments into a single, unified dataset. This integration enables a holistic view of each patient’s medical history and facilitates better care among healthcare providers.
Don’t let poor data drain your profits. Invest in clean data for enhanced returns.
Are you ready to get your data cleansed?
We have covered why data cleansing is necessary, the characteristics of high-quality data, and how you can achieve clean data. However, the process of cleansing requires attention to detail because even the slightest error can drastically affect your business. Therefore, it is advisable to either train your in-house team (still a significant cost and time investment on your part) or outsource data cleansing services to a third-party service provider for optimal results. You can weigh your options based on budget, data volume, and complexity and choose wisely.
Happy Cleansing!
FAQs
Q.1 What is data cleansing, and why is it essential for businesses?
Data cleansing is the process of identifying and correcting errors, inconsistencies, and inaccuracies in datasets. It ensures accurate decision-making, improved customer experiences, efficient operations, and better-targeted marketing campaigns.
Q.2 Can data cleansing improve data security and compliance?
Yes! Cleansing can enhance data security by identifying and removing sensitive or outdated information, reducing the risk of security breaches and unauthorized access. Additionally, it ensures data accuracy and validity, helping organizations adhere to regulatory requirements.
Q.3 How frequently should data cleansing be performed?
The frequency of data cleansing depends on factors like the rate of data accumulation, data source reliability, and business needs. Generally, it is recommended to perform data cleansing at frequent intervals, especially before critical analyses, reporting, or strategic decision-making.
Q.4 What are the benefits of outsourcing data cleansing services?
Outsourcing data cleansing services offers access to specialized expertise and saves considerable time and effort. It’s also a cost-effective solution, avoiding long-term overhead expenses. Reputable data cleansing companies use advanced technology, ensuring improved data quality for more informed decision-making. Additionally, data security and compliance are prioritized, assuring utmost confidentiality.